The future scope of machine learning: Top use cases
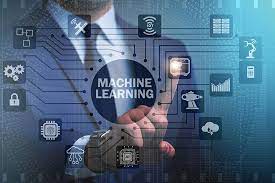
The future scope of machine learning: Top use cases
Machine learning is one of the best career options of the 21st century, with its broad future scope and high-paying, lucrative job opportunities. Before we delve into the future scope of machine learning, let’s get you introduced to machine learning. Machine learning teaches computers to make predictions or decisions using data without being explicitly programmed for a specific task. The computer learns from the data and improves its accuracy over time. In addition to machine learning, applied machine learning refers to using machine learning algorithms and models to solve practical, real-world problems in various domains, such as finance, healthcare, marketing, etc. It involves taking a problem, collecting and preprocessing data, selecting and training a model, and deploying it to make predictions or decisions. Applied machine learning aims to automate and improve decision-making processes using data-driven methods.
The future of machine learning is bright, with increasing demand for professionals with these skills. According to a recent report by Indeed, the number of job postings for machine learning skills has increased by 344% since 2013. In 2020, there was a 50% increase in demand for machine learning engineers compared to the previous year.
Another report by Gartner predicts that by 2022, 85% of customer interactions can be managed without a human, and machine learning will enable this change. Additionally, the market for machine learning is expected to grow from $1.03 billion in 2018 to $8.81 billion by 2022 at a compound annual growth rate (CAGR) of 44.1%. These statistics show that the demand for machine learning professionals and the market for machine learning solutions is only going to grow in the future, making it a promising field with a bright future.
Applied machine learning in Python refers to using the Python programming language and its various libraries and frameworks to develop and implement machine learning models to solve real-world problems.
Python has become a popular language for applied machine learning due to its ease of use, a large community of users and developers, and a rich set of libraries and tools such as NumPy, Pandas, Matplotlib, and sci-kit-learn. These libraries make it easier for practitioners to perform data manipulation, visualization, and model training and evaluation tasks. In applied machine learning in Python, students or practitioners learn to work with real-world data and use machine learning algorithms to build models that can make predictions, and classify, or cluster data into groups. The objective is to develop solutions that can automate decision-making and improve outcomes, making the most of the data available.
Table of Contents
Top Use Cases of Machine Learning
To better understand the importance and future scope of machine learning, we’ve explained its top use cases below:
Supply Chain
Machine learning is increasingly being applied in supply chain management to improve efficiency and optimize operations. Applications include demand forecasting, which uses historical data and machine learning algorithms to predict future demand for products, enabling better inventory management. Another example is supplier selection and evaluation, which involves analyzing supplier performance, costs, and delivery times to identify the best suppliers.
Additionally, machine learning can be used for anomaly detection in supply chain processes, helping to identify and prevent issues such as production bottlenecks, delivery delays, and quality control problems. Overall, machine learning in supply chain management helps organizations make better decisions, improve visibility, and increase responsiveness to changing market conditions, leading to increased efficiency, lower costs, and improved customer satisfaction.
Product Development
Machine learning is applied in product development to accelerate the design process and improve product quality. Applications include product design optimization, which uses machine learning algorithms to identify the best design parameters for a product based on factors such as cost, performance, and customer preferences. Another example is computer-aided design, which uses machine learning to assist designers in creating and simulating new product designs.
Machine learning can also be used to analyze customer feedback and sales data, providing valuable insights into customer preferences and market trends and helping to inform future product development decisions. With the ability to process enormous amounts of data and identify patterns and relationships, machine learning can transform product development, enabling companies to design better products faster and at lower costs. Overall, machine learning in product development helps organizations stay ahead of the curve, improve product quality, and meet customer demands more effectively.
Autonomous Vehicles
Machine learning plays a critical role in the development of autonomous vehicles. It is used for various tasks, including object detection and classification, lane detection, and vehicle behavior prediction. For example, machine learning algorithms can analyze data from cameras, lidar, and radar sensors to identify objects on the road and make decisions about how to navigate around them safely.
Additionally, machine learning can improve the accuracy of self-driving algorithms over time through reinforcement learning, which allows the vehicle to learn from its experiences and continually improve its performance. Machine learning also enables vehicles to anticipate and respond to changing traffic patterns and road conditions, making autonomous driving safer and more efficient. By allowing vehicles to process vast amounts of data and make complex decisions in real-time, machine learning can revolutionize the field of autonomous vehicles, making it possible for vehicles to drive themselves safely and reliably.
Image Recognition
Machine learning is widely used in image recognition, which involves automatically classifying objects, people, and scenes in digital images. Machine learning algorithms can analyze and learn from large datasets of images and then use that knowledge to identify objects in new images. Image recognition applications include facial recognition, which is used for security purposes and in social media platforms to tag people in photos.
Another example is object detection, which can be used in retail, transportation, and healthcare industries to automatically identify and track specific objects. Additionally, machine learning can be used for image classification, which involves assigning images to predefined categories, such as animals, landscapes, or food. Machine learning in image recognition can revolutionize industries and make our lives easier, faster, and more convenient by automating tasks previously performed manually.
Conclusion:
The future scope of applied machine learning courses is immense and holds great potential for businesses and industries. From healthcare and finance to retail and transportation, machine learning has already begun to transform how we live and work, and this trend will continue in the coming years. With the ability to process massive amounts of data and make decisions based on that data, machine learning has the potential to drive significant improvements in efficiency, accuracy, and productivity. As machine learning algorithms evolve and become more advanced, new and innovative use cases will likely emerge, driving further growth and development in this exciting field. The future of machine learning is bright and holds endless possibilities for businesses and individuals alike